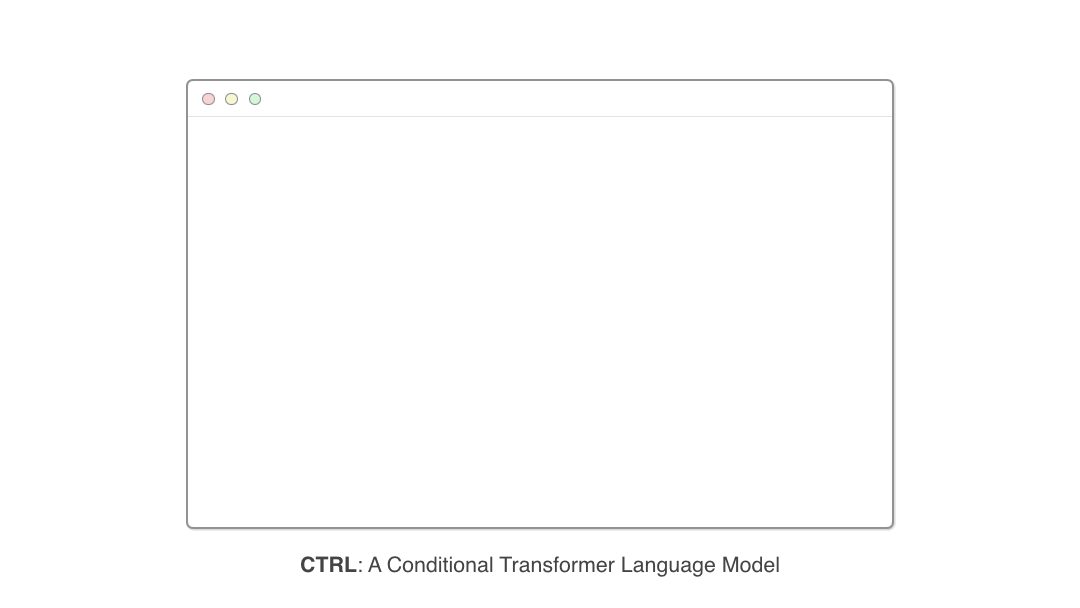
Introduction
In the ever-evolving landscape of natural language processing (NLP), the quest for more refined control over text generation has led to the development of CTRL, a state-of-the-art language model by Salesforce. My work at salesforce, has offered me a unique perspective on its significance and the technological strides it represents. Through this article, I aim to distill the essence of our work on CTRL, making the complex world of AI more accessible and understandable.
Introduction to CTRL
CTRL stands for Conditional Transformer Language Model, a machine learning model designed to generate text based on specific user inputs, known as control codes. With a whopping 1.63 billion parameters, CTRL stands out in its ability to manipulate various aspects of generated text, such as style, content, and domain-specific characteristics. What sets CTRL apart is its training process, which leverages control codes derived from the inherent structure found within vast amounts of text data. This approach not only retains the benefits of unsupervised learning but also introduces a higher degree of explicit control for users over the generated text.
The Data Behind the Intelligence
The backbone of CTRL's exceptional capabilities is the diverse dataset it was trained on, encompassing 140 GB of text from various domains such as Wikipedia, Project Gutenberg, numerous subreddits, OpenWebText, a comprehensive collection of news data, Amazon Reviews, and many more. This eclectic dataset enabled the model to understand and generate text across a wide range of topics and styles, making it incredibly versatile.
Innovations in Text Generation
One of the core advancements brought about by CTRL is its novel approach to sampling, which is crucial for text generation. Traditional models often struggle with finding a balance between creativity and coherence, but CTRL introduces a sampling mechanism that trusts the model's distribution while mitigating repetitive patterns. This is achieved through penalized sampling, which cleverly discounts the scores of previously generated tokens, thus encouraging diversity without sacrificing accuracy.
Furthermore, CTRL's use of control codes is a game-changer. These codes enable users to guide the model's output more precisely, dictating not only the general style but also specific details like domain, entity relations, and even task-specific instructions. Whether it's generating text in a particular literary style or answering complex questions, CTRL's control codes unlock a new level of specificity in text generation.
The Future of Text Generation
Looking ahead, the potential for further refining and expanding the control mechanisms within CTRL is vast. The project hints at future directions where even more nuanced control codes could be developed, leveraging the natural structure of the internet and specific data attributes for unparalleled precision in text generation. Moreover, the implications of CTRL extend beyond text generation to other areas of NLP, promising enhancements in tasks like machine translation and question answering.
Conclusion
The release of CTRL represents a significant milestone in the quest for more controllable and versatile language models. With its sophisticated architecture and innovative use of control codes, CTRL not only showcases the potential of controlled text generation but also sets the stage for future advancements in the field. As a minor contributor to this groundbreaking project, I've witnessed firsthand the dedication and ingenuity that went into making CTRL a reality. It's an exciting time in the world of NLP, and CTRL is undoubtedly leading the charge towards a future where humans can interact with AI in more meaningful and precise ways.